Sub-Saharan Africa will increasingly become the dominant hotspot of surface water pollution
Human activities greatly impact surface water quality, while being reliant upon it for water supply. Surface water quality is expected to change in the future as a result of alterations to pollutant loadings, surface water withdrawals and hydrological regimes, driven by both climate change and socio-economic developments. Here we use a high-resolution global surface water quality model to project water temperature and indicators of salinity (total dissolved solids), organic (biological oxygen demand) and pathogen (fecal coliform) pollution until 2100. The results show that while surface water quality, as indicated by these pollutants, will improve in most advanced economies, the outlook for poorer nations is bleak. The proportion of the global population exposed to salinity, organic and pathogen pollution by the end of the century ranges from 17 to 27%, 20 to 37% and 22 to 44%, respectively, with poor surface water quality disproportionately affecting people living in developing countries. Exhibiting the largest increases in both the absolute and relative number of people exposed, irrespective of climate change and socio-economic development scenario, we conclude that Sub-Saharan Africa will become the new hotspot of surface water pollution globally.
This is a preview of subscription content, access via your institution
Access options
Subscribe to this journal
Receive 12 digital issues and online access to articles
133,45 € per year
only 11,12 € per issue
Buy this article
- Purchase on SpringerLink
- Instant access to full article PDF
Prices may be subject to local taxes which are calculated during checkout
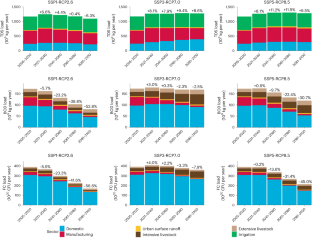
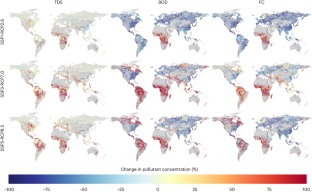
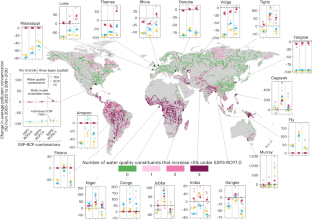
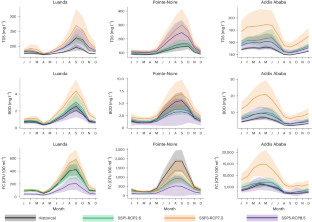

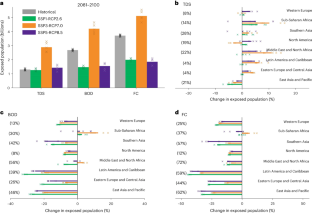
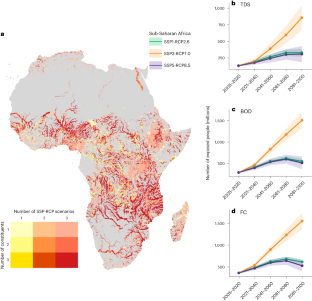
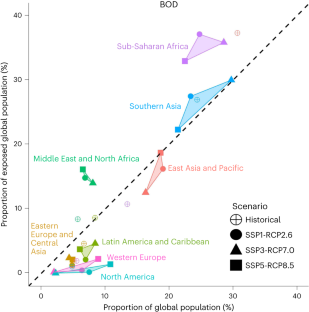
Similar content being viewed by others
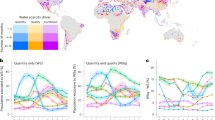
Current and future global water scarcity intensifies when accounting for surface water quality
Article 23 May 2024
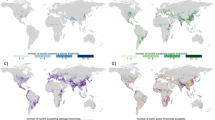
Current wastewater treatment targets are insufficient to protect surface water quality
Article Open access 06 October 2022
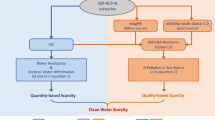
A triple increase in global river basins with water scarcity due to future pollution
Article Open access 06 February 2024
Data availability
Global hydrological and surface water quality output at 10 km resolution, per GCM and global change scenario (RCP–SSP), is available open-access at https://doi.org/10.5281/zenodo.7811612.
Code availability
The surface water quality model used in this study, DynQual, is available open-access through a GitHub repository (https://github.com/UU-Hydro/DYNQUAL).
References
- Arnell, N. W. Climate change and global water resources. Global Environ. Change9, S31–S49 (1999). ArticleGoogle Scholar
- Haddeland, I. et al. Global water resources affected by human interventions and climate change. Proc. Natl Acad. Sci. USA111, 3251–3256 (2014). ArticleCASPubMedGoogle Scholar
- Konapala, G., Mishra, A. K., Wada, Y. & Mann, M. E. Climate change will affect global water availability through compounding changes in seasonal precipitation and evaporation. Nat. Commun.11, 3044 (2020). ArticleCASPubMedPubMed CentralGoogle Scholar
- Kraaijenbrink, P. D. A., Stigter, E. E., Yao, T. & Immerzeel, W. W. Climate change decisive for Asia’s snow meltwater supply. Nat. Clim. Change11, 591–597 (2021). ArticleGoogle Scholar
- Trenberth, K. E. et al. Global warming and changes in drought. Nat. Clim. Change4, 17–22 (2014). ArticleGoogle Scholar
- Wada, Y. et al. Modeling global water use for the 21st century: the Water Futures and Solutions (WFaS) initiative and its approaches. Geosci. Model Dev.9, 175–222 (2016). ArticleGoogle Scholar
- Jones, E. R. et al. Current wastewater treatment targets are insufficient to protect surface water quality. Commun. Earth Environ.3, 221 (2022). ArticleGoogle Scholar
- van Vliet, M. T. H. et al. Global water scarcity including surface water quality and expansions of clean water technologies. Environ. Res. Lett.16, 024020 (2021). ArticleGoogle Scholar
- Jones, E. R. et al. DynQual v1.0: a high-resolution global surface water quality model. Geosci. Model Dev. Discuss.2022, 1–24 (2022). Google Scholar
- Jones, E. R., van Vliet, M. T. H., Qadir, M. & Bierkens, M. F. P. Country-level and gridded estimates of wastewater production, collection, treatment and reuse. Earth Syst. Sci. Data13, 237–254 (2021). ArticleGoogle Scholar
- Bosmans, J. et al. FutureStreams, a global dataset of future streamflow and water temperature. Sci. Data9, 307 (2022). ArticlePubMedPubMed CentralGoogle Scholar
- Reder, K., Flörke, M. & Alcamo, J. Modeling historical fecal coliform loadings to large European rivers and resulting in-stream concentrations. Environ. Model. Softw.63, 251–263 (2015). ArticleGoogle Scholar
- Caretta, M. A. et al. in Climate Change 2022: Impacts, Adaptation, and Vulnerability. Contribution of Working Group II to the Sixth Assessment Report of the Intergovernmental Panel on Climate Change. (eds H.-O. Pörtner, D.C. Roberts, M. Tignor, E.S. Poloczanska, K. Mintenbeck, A. Alegría, M. Craig, S. Langsdorf, S. Löschke, V. Möller, A. Okem, B. Rama) pp. 551–712 (Cambridge Univ. Press, 2022).
- Schewe, J. et al. Multimodel assessment of water scarcity under climate change. Proc. Natl Acad. Sci. USA111, 3245–3250 (2014). ArticleCASPubMedGoogle Scholar
- Riahi, K. et al. The Shared Socioeconomic Pathways and their energy, land use, and greenhouse gas emissions implications: an overview. Global Environ. Change42, 153–168 (2017). ArticleGoogle Scholar
- O’Neill, B. C. et al. The roads ahead: narratives for shared socioeconomic pathways describing world futures in the 21st century. Global Environ. Change42, 169–180 (2017). ArticleGoogle Scholar
- Wada, Y., Wisser, D. & Bierkens, M. F. P. Global modeling of withdrawal, allocation and consumptive use of surface water and groundwater resources. Earth Syst. Dynam.5, 15–40 (2014). ArticleGoogle Scholar
- Döll, P. et al. Risks for the global freshwater system at 1.5 °C and 2 °C global warming. Environ. Res. Lett.13, 044038 (2018). ArticleGoogle Scholar
- Hanasaki, N., Yoshikawa, S., Pokhrel, Y. & Kanae, S. A quantitative investigation of the thresholds for two conventional water scarcity indicators using a state-of-the-art global hydrological model with human activities. Water Resour. Res.54, 8279–8294 (2018). ArticleGoogle Scholar
- van Vliet, M. et al. Global river discharge and water temperature under climate change. Global Environ. Change23, 450–464 (2013). ArticleGoogle Scholar
- Sutanudjaja, E. et al. PCR-GLOBWB 2: a 5 arcmin global hydrological and water resources model. Geosci. Model Dev.11, 2429–2453 (2018). ArticleGoogle Scholar
- UNEP. A snapshot of the world’s water quality: towards a global assessment. United Nations Environment Programme, Nairobi, Kenyahttps://www.unep.org/resources/publication/snapshot-report-worlds-water-quality (2016).
- Büchner, S. L. A. ISIMIP3b bias-adjusted atmospheric climate input data. ISIMIPhttps://doi.org/10.48364/ISIMIP.842396.1 (2021).
- Hoch, J. M., Sutanudjaja, E. H., Wanders, N., van Beek, R. L. P. H. & Bierkens, M. F. P. Hyper-resolution PCR-GLOBWB: opportunities and challenges from refining model spatial resolution to 1 km over the European continent. Hydrol. Earth Syst. Sci.27, 1383–1401 (2023). ArticleGoogle Scholar
- Wanders, N., van Vliet, M. T. H., Wada, Y., Bierkens, M. F. P. & van Beek, L. P. H. High-resolution global water temperature modeling. Water Resour. Res.55, 2760–2778 (2019). ArticleGoogle Scholar
- Her, Y. et al. Uncertainty in hydrological analysis of climate change: multi-parameter vs. multi-GCM ensemble predictions. Sci. Rep.9, 4974 (2019). ArticlePubMedPubMed CentralGoogle Scholar
- van der Wiel, K., Wanders, N., Selten, F. M. & Bierkens, M. F. P. Added value of large ensemble simulations for assessing extreme river discharge in a 2 °C warmer world. Geophys. Res. Lett.46, 2093–2102 (2019). ArticleGoogle Scholar
- Damania, R., Desbureaux, S., Rodella, A.-S., Russ, J. & Zaveri, E. Quality Unknown: the Invisible Water Crises (World Bank Group, 2019). BookGoogle Scholar
- Barbarossa, V. et al. Threats of global warming to the world’s freshwater fishes. Nat. Commun.12, 1701 (2021). ArticleCASPubMedPubMed CentralGoogle Scholar
- Wen, Y., Schoups, G. & van de Giesen, N. Organic pollution of rivers: combined threats of urbanization, livestock farming and global climate change. Sci. Rep.7, 43289 (2017). ArticleCASPubMedPubMed CentralGoogle Scholar
- Desbureaux, S. et al. Mapping global hotspots and trends of water quality (1992–2010): a data driven approach. Environ. Res. Lett.17, 114048 (2022). ArticleGoogle Scholar
- GEMStat database of the Global Environment Monitoring System for Freshwater (GEMS/Water) Programme (UNEP, 2020); https://gemstat.org/data/data-portal/
- Beusen, A. H. W. et al. Exploring river nitrogen and phosphorus loading and export to global coastal waters in the Shared Socio-economic pathways. Global Environ. Change72, 102426 (2022). ArticleGoogle Scholar
- Xie, H. & Ringler, C. Agricultural nutrient loadings to the freshwater environment: the role of climate change and socioeconomic change. Environ. Res. Lett.12, 104008 (2017). ArticleGoogle Scholar
- Morée, A. L., Beusen, A. H. W., Bouwman, A. F. & Willems, W. J. Exploring global nitrogen and phosphorus flows in urban wastes during the twentieth century. Global Biogeochem. Cycles27, 836–846 (2013). ArticleGoogle Scholar
- Van Drecht, G., Bouwman, A. F., Harrison, J. & Knoop, J. M. Global nitrogen and phosphate in urban wastewater for the period 1970 to 2050. Glob. Biogeochem. Cycleshttps://doi.org/10.1029/2009gb003458 (2009).
- Jones, B. & O’Neill, B. C. Spatially explicit global population scenarios consistent with the Shared Socioeconomic Pathways. Environ. Res. Lett.11, 084003 (2016). ArticleGoogle Scholar
- Hurtt, G. C. et al. Harmonization of global land use change and management for the period 850–2100 (LUH2) for CMIP6. Geosci. Model Dev.13, 5425–5464 (2020). ArticleCASGoogle Scholar
- Gilbert, M. et al. Global distribution data for cattle, buffaloes, horses, sheep, goats, pigs, chickens and ducks in 2010. Sci. Data5, 180227 (2018). ArticlePubMedPubMed CentralGoogle Scholar
- Graham, N. T. et al. Water sector assumptions for the Shared Socioeconomic Pathways in an integrated modeling framework. Water Resour. Res.54, 6423–6440 (2018). ArticleGoogle Scholar
- Lohrmann, A., Farfan, J., Caldera, U., Lohrmann, C. & Breyer, C. Global scenarios for significant water use reduction in thermal power plants based on cooling water demand estimation using satellite imagery. Nat. Energy4, 1040–1048 (2019). ArticleGoogle Scholar
- van Puijenbroek, P. J. T. M., Beusen, A. H. W. & Bouwman, A. F. Global nitrogen and phosphorus in urban waste water based on the Shared Socio-economic pathways. J. Environ. Manage.231, 446–456 (2019). ArticlePubMedGoogle Scholar
- Jones, E., Qadir, M., van Vliet, M. T. H., Smakhtin, V. & Kang, S.-M. The state of desalination and brine production: a global outlook. Sci. Total Environ.657, 1343–1356 (2019). ArticleCASPubMedGoogle Scholar
- Fick, S. E. & Hijmans, R. J. WorldClim 2: new 1-km spatial resolution climate surfaces for global land areas. Int. J. Climatol.37, 4302–4315 (2017). ArticleGoogle Scholar
- Fipps, G. Irrigation water quality standards and salinity management strategies. Texas FARMER Collectionhttps://twon.tamu.edu/wp-content/uploads/sites/3/2021/06/irrigation-water-quality-standards-and-salinity-management-strategies-1.pdf (2003).
- Zaman, M., Shahid, S. A. & Heng, L. in Guideline for Salinity Assessment, Mitigation and Adaptation Using Nuclear and Related Techniques 113–131 (Springer International Publishing, 2018).
- Nahian, M. A. et al. Drinking water salinity associated health crisis in coastal Bangladesh. Elementa Sci Anthr.https://doi.org/10.1525/elementa.143 (2018).
Acknowledgements
M.T.H.v.V. was financially supported by the Netherlands Scientific Organisation (NWO) by a VIDI grant (VI.Vidi.193.019) and European Research Council under the European Union’s Horizon Europe research and innovation programme (grant agreement 101039426). We acknowledge and thank the Netherlands Organisation for Scientific Research (NWO) for the grant that enabled us to use the national supercomputer Snellius (project: EINF-3999).
Author information
Authors and Affiliations
- Department of Physical Geography, Faculty of Geosciences, Utrecht University, Utrecht, the Netherlands Edward R. Jones, Marc F. P. Bierkens, Ludovicus (Rens) P. H. van Beek, Niko Wanders, Edwin H. Sutanudjaja & Michelle T. H. van Vliet
- Deltares, Unit Soil and Groundwater Systems, Utrecht, the Netherlands Marc F. P. Bierkens
- PBL Netherlands Environmental Assessment Agency, The Hague, the Netherlands Peter J. T. M. van Puijenbroek
- Edward R. Jones